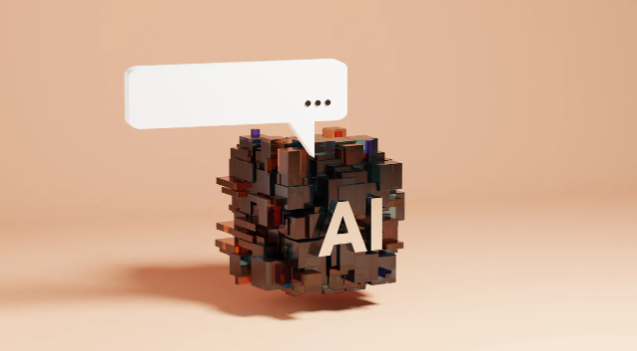
What is generative AI?
Generative artificial intelligence (AI) refers to models or algorithms that create brand-new output, such as text, photos, videos, code, data, or 3D renderings, from the huge amount of data they are trained on. The models ‘generate’ new content by referring to the data they have been trained on, making new predictions.
The purpose of generative AI is to create content, as opposed to other forms of AI, which suit different purposes, such as analyzing data, making ad recommendations, parsing through applications, helping to control a self-driving car, etc.
What is an example of generative AI?
As mentioned above, generative AI is simply a subsection of AI that uses its training data to ‘generate’ or produce a new output. AI chatbots or AI image generators are quintessential examples of generative AI models. These tools use vast amounts of materials they were trained on to create new text or images.
Why is generative AI a hot topic right now?
The term generative AI is causing a buzz because of the increasing popularity of generative AI models, such as OpenAI’s conversational chatbot ChatGPT and its AI image generator DALL-E 3.
These and similar tools use generative AI to produce new content, including computer code, essays, emails, social media captions, images, poems, Excel formulas, and more, within seconds, which has the potential to boost peoples’ workflows significantly.
ChatGPT became extremely popular quickly, accumulating over one million users a week after launching. Many other companies saw that success and rushed to compete in the generative AI marketplace, including Google, Microsoft’s Bing, and Anthropic. These companies quickly developed their own generative AI models.
The buzz around generative AI will keep growing as more companies enter the market and find new use cases to help the technology integrate into everyday processes. For example, there has been a recent surge of new generative AI models for video and audio.
What does machine learning have to do with generative AI?
Machine learning refers to the subsection of AI that teaches a system to make a prediction based on data it’s trained on. An example of this prediction is when DALL-E 3 creates an image based on the prompt you enter by discerning what the prompt means.
Generative AI is, therefore, a machine-learning framework, but all machine-learning frameworks are not generative AI.
What is the difference between generative AI and LLM?
When discussing generative AI models, you often hear the term large language model (LLM) because it is the technology that powers AI chatbots.
As ZDNET’s Maria Diaz explains: “One of the most renowned types of AI right now is large language models (LLM). These models use unsupervised machine learning and are trained on massive amounts of text to learn how human language works. These texts include articles, books, websites, and more.”
These LLMs have advanced natural language processing abilities and are often used for AI chatbots. These chatbots need to understand conversational prompts from users, but they also need to output prompts conversationally.
Some of the most popular LLMs are OpenAI’s GPT-3.5, which powers the free version of ChatGPT, and GPT-4, which powers ChatGPT Plus and Microsoft’s Copilot.
What are text-based generative AI models trained on?
Text-based models, such as ChatGPT, are trained on massive amounts of data in a process known as self-supervised learning. Here, the model learns from the information it’s fed to make predictions and generate answers in future scenarios
One concern with generative AI models, especially those that generate text, is that many are trained on data from the entirety of the internet. This data includes copyrighted material and information that might not have been shared with the owner’s consent.
What is generative AI art?
Generative AI art, including images, is created by AI models trained on billions of images. The model uses this data to learn styles of pictures and then uses this insight to generate new art when prompted by an individual through text.
A popular example of an AI art generator is DALL-E. However, plenty of other AI generators are on the market and are just as good, if not more capable. These tools can also be used for different requirements.
Image Creator from Microsoft Designer is Microsoft’s take on the technology, which leverages OpenAI’s most advanced text-to-image model, DALL-E 3, and is currently viewed by ZDNET as the best AI image generator.
Some models, such as DALL-E, are trained with images found across the internet, even if the creator’s permission wasn’t granted. Others, such as Adobe’s Firefly, take a more ethical approach, reportedly using only Adobe Stock Images or public domain content where the copyright has expired.
What are the problems with art generated by text-to-image models?
Many generative AI art models are trained on billions of images from the internet. This content often includes artwork and images produced by artists and creatives. These images are then reimagined and repurposed by AI to generate your image. The catch is that the artists of the original work did not consent to their artwork being used to train AI models and inspire others.
Although it’s not the same image, the new image has elements of an artist’s original work, which is not credited to them. A specific style unique to the artist can be replicated by AI and used to generate a new image, without the original artist knowing or approving. The debate about whether AI-generated art is ‘new’ or even ‘art’ will continue for many years.
What are some shortcomings of generative AI?
Generative AI models take a vast amount of content from across the internet and then use the information they are trained on to make predictions and create an output for the prompts you input. These predictions are based on the data the models are fed, but there are no guarantees the prediction will be correct, even if the responses sound plausible.
The responses might also incorporate biases inherent in the content the model has ingested from the internet, but there is often no way of knowing whether that’s true. These shortcomings have caused major concerns regarding the spread of misinformation due to generative AI.
Generative AI models don’t necessarily know whether their output is accurate. Users are unlikely to know where information has come from. They are also unlikely to understand how the algorithms process data to generate content.
There are examples of chatbots providing incorrect information or simply making things up to fill the gaps. While the results from generative AI can be intriguing and entertaining, it would be unwise, certainly in the short term, to rely on the information or content they create.
Some generative AI models, such as Copilot, are attempting to bridge that source gap by providing footnotes with sources that enable users to understand where their response comes from and verify its accuracy.